Implementation of data analytics tasks to improve the quality of services in the communications networks
Keywords:
data analytics, semantic mining, data mining, telecomunications, QoSAbstract
This work specifies Autonomous Cycles (AC) of data analysis tasks, to optimize the Quality Of Services (QoS) on the Internet. The mechanisms to improve QoS on the Internet are important for Internet Service Providers (ISP). These mechanisms should be based on context analysis, Deep Packet Inspection (DPI), the use of data mining and semantics, among others. The ACs of data analysis proposed in this work integrate these aspects, to perform tasks to improve QoS on the Internet, such as the task of classifying traffic on the network. In this paper the MIDANO methodology is used, to specify the two ACs that are proposed, one with the aim of improving the QoS on the Internet, and another with the objective of learning the traffic pattern in the network. In addition, this work implements the AC that improves the QoS on the internet. This AC monitors the state of Internet traffic, determines the behavior of applications, characterizes traffic patterns, generates traffic optimization rules, among other things, using DPI techniques, semantic mining, machine learning, among others.
Downloads
References
J. Aguilar; O. Buendia; K. Moreno; D. Mosquera. Autonomous cycle of data analysis tasks for learning processes. In Second International Conference on Technologies and Innovation (CITI), pages 187–202, Guayaquil, Ecuador, 2016. Springer International Publishing.
T. Nguyen; G. Armitage. A survey of techniques for internet traffic classification using machine learning. IEEE Communications Surveys & Tutorials, 10(4):56–76, 2008.
M. Soysal; E. Schmidt. Machine learning algorithms for accurate flow-based network traffic classification: Evaluation and comparison. Performance Evaluation, 67(6):451–467, 2010.
M. Roughan; S. Sen; O. Spatscheck; N. Duffield. Class-of-service mapping for qos: a statistical signature-based approach to ip traffic classification.In 4th ACM SIGCOMM Conference on Internet measurement, pages 135–148, New York, USA, 2004. ACM.
R. Sommer; V. Paxson. Outside the closed world: On using machine learning for network intrusion detection. In Security and Privacy, IEEE Symposium on, pages 305–316. IEEE, 2010.
D. Le; N. Zincir-Heywood; M. Heywood. Data analytics on network traffic flows for botnet behaviour detection. In Computational Intelligence (SSCI), IEEE Symposium Series on, pages 1–7. IEEE, 2016.
J. Aguilar; O. Buendía; J. Cordero. Specification of the autonomic cycles of learning analytic tasks for a smart classroom. Journal of Educational Computing Research, 2017.
F. Pacheco; J. Aguilar; C. Rangel; M. Cerrada; J. Altamiranda. Methodological framework for data processing based on the data science paradigm. In Computing Conference (CLEI), XL Latin American, pages 1–12. IEEE, 2014.
C. Rangel; F. Pacheco; J. Aguilar; M. Cerrada; J. Altamiranda. Methodology for detecting the feasibility of using data mining in an organization. In Computing Conference (CLEI), 2013 XXXIX Latin American, pages 502–513. IEEE, 2013.
G. Riofrio; E. Encalada; D. Guamán; J. Aguilar. Business intelligence applied to learning analytics in student-centered learning processes. In Computing Conference (CLEI), Latin American, pages 567–576. IEEE, 2014.
Tweepy API. Libreria python para accesar la api de twitter. http://www.tweepy.org/, Última consulta 26 de mayo de 2018.
R. Goss; R. Botha. Establishing discernible flow characteristics for accurate, real-time network protocol identification. In INC, pages 25–34, 2012.
Y. Hadjadj-Aoul; K. Singh; S. Vaton; M. Sbai; S. Moteau. Technical specifications of the modules proposed within the wp2: Traffic classifier, qoe evaluator and their integration, technical report, projet vipeer ingénierie du trafic vidéo en intradomaine basèe sur les paradigmes du pair à pair. 2011.
M. Crotti; F. Gringoli; P. Pelosato; L. Salgarelli. A statistical approach to ip-level classification of network traffic. In Communications, ICC’06.IEEE International Conference on, volume 1, pages 170–176. IEEE, 2006.
V. Paxson. Empirically derived analytic models of wide-area tcp connections. IEEE/ACM Transactions on Networking (TON), 2(4):316–336,
T. Karagiannis; K. Papagiannaki; M. Faloutsos. Blinc: multilevel traffic classification in the dark. In ACM SIGCOMM computer communication review, volume 35, pages 229–240. ACM, 2005.
C. Dewes; A.Wichmann; A. Feldmann. An analysis of internet chat systems. In Proceedings of the 3rd ACM SIGCOMM conference on Internet measurement, pages 51–64. ACM, 2003.
A. Arcia-Moret; A. Araujo; J. Aguilar; L. Molina; A. Sathiaseelan. Intelligent network discovery for next generation community wireless networks. In 12th conference on Wireless On-demand Network systems and Services Conference, pages 81–87, Italy, 2016. IEEE.
H. Kim; K. Claffy; M. Fomenkov; D. Barman; M. Faloutsos; K. Lee. Internet traffic classification demystified: myths, caveats, and the best practices. In Proceedings of the 2008 ACM CoNEXT conference, page 11. ACM, 2008.
A. Arcia-Moret; A. Sathiaseela; A. Araujo; J. Aguilar; L. Molina. Assisted network discovery for next generation wireless networks. In Consumer Communications & Networking Conference (CCNC), 13th IEEE Annual, pages 800–801. IEEE, 2016.
A. Moore; D. Zuev. Internet traffic classification using bayesian analysis techniques. In ACM SIGMETRICS Performance Evaluation Review, volume 33, pages 50–60. ACM, 2005.
D. Nechay. Controlling False Alarm/Discovery Rates in Online Internet Traffic Classification. PhD thesis, McGill University, 2009.
J. Tan; X. Chen; M. Du; K. Zhu. A novel internet traffic identification approach using wavelet packet decomposition and neural network. Journal of Central South University, 19(8):2218–2230, 2012.
B. Yamansavascilar; M. Guvensan; A. Yavuz; M. Karsligil. Application identification via network traffic classification. In Computing, Networking and Communications (ICNC),International Conference on, pages 843–848. IEEE, 2017.
J. Tan; X. Chen; M. Du. An internet traffic identification approach based on ga and pso-svm. JCP, 7(1):19–29, 2012.
U. Trivedi; M. Patel. A fully automated deep packet inspection verification system with machine learning. In Advanced Networks and Telecommunications Systems (ANTS), IEEE International Conference on, pages 1–6. IEEE, 2016.
M. Shafiq; X. Yu; A. Laghari; L. Yao; N. Karn; F. Abdesssamia. Wechat text and picture messages service flow traffic classification using machine learning technique. In High Performance Computing and Communications; IEEE 14th International Conference on Smart City; IEEE 2nd International Conference on Data Science and Systems (HPCC/SmartCity/DSS), IEEE 18th International Conference on, pages 58–62.IEEE, 2016.
M. Shafiq; X. Yu; A. Laghari; L. Yao; N. Karn; F. Abdessamia. Network traffic classification techniques and comparative analysis using machine learning algorithms. In Computer and Communications (ICCC), 2nd IEEE International Conference on, pages 2451–2455. IEEE, 2016.
L. Caviglione; N. Celandroni; M. Collina; H. Cruickshank; G. Fairhurst; E. Ferro; A. Gotta; M. Luglio; C. Roseti; A. Abdel Salam; A. Vanelli. A deep analysis on future web technologies and protocols over broadband geo satellite networks. International Journal of Satellite Communications and Networking, 33(5):451–472, 2015.
K. Alhamazani; R. Ranjan; P. Jayaraman; K. Mitra; M. Wang; Z. Huang; L.Wang; F. Rabhi. Real-time qos monitoring for cloud-based big data analytics applications in mobile environments. In Mobile Data Management (MDM), IEEE 15th International Conference on, volume 1, pages 337–340. IEEE, 2014.
Pace: Dpi engine. https://www.ipoque.com/en/products/pace, Ultima consulta 26 de mayo de 2018.
SolarWinds. Solarwinds network performance monitor. http://www.solarwinds.com/network-performance-monitor, Ultima consulta 26 de mayo de 2018.
NDPI: herramienta de dpi. https://github.com/ntop/nDPI/issues/249, Última consulta 26 de mayo de 2018.
L. Deri; M. Martinelli; T. Bujlow; A. Cardigliano. ndpi: Open-source high-speed deep packet inspection. In Wireless Communications and Mobile Computing Conference (IWCMC), International, pages 617–622. IEEE, 2014.
P. C. Lin; Y. D. Lin; Y. C. Lai; T. H. Lee. Using string matching for deep packet inspection. Computer, 41(4):23, 2008.
K. Kyriakopoulos; D. Parish; J. Whitley. Flowstats: An ontology based network management tool. In Computing Technology and Information Management (ICCTIM), Second International Conference on, pages 13–18. IEEE, 2015.
Pandas API. Libreria de python para analítica de datos. http://pandas.pydata.org/, Ultima consulta 26 de mayo de 2018.
Wireshark project. Analizador de paquetes de red gratuito y abierto. https://www.wireshark.org/, Ultima consulta 26 de mayo de 2018.
Weka 3: Data mining software in java. https://www.cs.waikato.ac.nz/ml/weka/, Última consulta 26 de mayo de 2018.
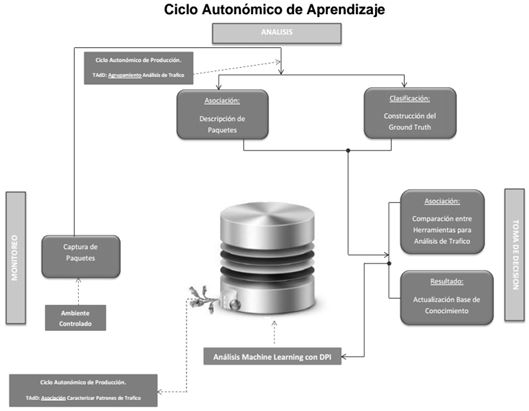
Published
How to Cite
Issue
Section
Creative Commons Reconocimiento-NoComercial-CompartirIgual 4.0 Internacional (CC BY-NC-SA 4.0)
The opinions expressed by the authors do not necessarily reflect the position of the publisher of the publication or of UCLA. The total or partial reproduction of the texts published here is authorized, as long as the complete source and the electronic address of this journal are cited.
The authors fully retain the rights to their works, giving the journal the right to be the first publication where the article is presented. The authors have the right to use their articles for any purpose as long as it is done for non-profit. Authors are recommended to disseminate their articles in the final version, after publication in this journal, in the electronic media of the institutions to which they are affiliated or personal digital media.